- Xinwei Networks Network & Wireless Cards Driver Download For Windows Xp
- Xinwei Networks Network & Wireless Cards Driver Download For Windows 7
- Xinwei Networks Network & Wireless Cards Driver Download For Windows 10
- Xinwei Networks Network & Wireless Cards Driver Download For Windows 8

Beijing Xinwei Yachen Network Information Service Co., Ltd. (Xinwei Yachen), founded in February 2012 with a registered capital of 200 million RMB, is a company devoted to the construction and operation of Xinwei domestic wireless government affairs networks. Xinwei Deng is an Associate Profoessor of Statistics at Virginia Tech. He is also a co-director of VT Statistics and Artificial Intelligence Laboratory.Deng obtained his Ph.D. Degree from Georgia Institute of Techonology in 2009 under the supervison of Jeff Wu and Ming Yuan. 2 illustrates the effect of regularization factors on network steady-state MSD for all competing algorithms. As we can notice from right-top, the steady-state MSD can be below -21 dB if values of (γ 1, γ 2) are properly chosen in the fused-ZA-ATC algorithm, while the minimal steady-state MSD is only around -16.15 dB in the ZA-ATC algorithm, which indicates that the fused-ZA-ATC.
Download PDFAbstract: Heterogeneous information networks (HINs) with rich semantics are ubiquitousin real-world applications. For a given HIN, many reasonable clustering resultswith distinct semantic meaning can simultaneously exist. User-guided clusteringis hence of great practical value for HINs where users provide labels to asmall portion of nodes. To cater to a broad spectrum of user guidance evidencedby different expected clustering results, carefully exploiting the signalsresiding in the data is potentially useful. Meanwhile, as one type of complexnetworks, HINs often encapsulate higher-order interactions that reflect theinterlocked nature among nodes and edges. Network motifs, sometimes referred toas meta-graphs, have been used as tools to capture such higher-orderinteractions and reveal the many different semantics. We therefore approach theproblem of user-guided clustering in HINs with network motifs. In this process,we identify the utility and importance of directly modeling higher-orderinteractions without collapsing them to pairwise interactions. To achieve this,we comprehensively transcribe the higher-order interaction signals to a seriesof tensors via motifs and propose the MoCHIN model based on joint non-negativetensor factorization. This approach applies to arbitrarily many, arbitraryforms of HIN motifs. An inference algorithm with speed-up methods is alsoproposed to tackle the challenge that tensor size grows exponentially as thenumber of nodes in a motif increases. We validate the effectiveness of theproposed method on two real-world datasets and three tasks, and MoCHINoutperforms all baselines in three evaluation tasks under three differentmetrics. Additional experiments demonstrated the utility of motifs and thebenefit of directly modeling higher-order information especially when userguidance is limited.
Submission history
From: Yu Shi [view email][v1] Wed, 28 Nov 2018 00:16:03 UTC (614 KB)
[v2] Thu, 27 Jun 2019 02:51:29 UTC (1,509 KB)

Full-text links:
Download:
Current browse context:
References & Citations
DBLP - CS Bibliography
Xinwei He
Naijing Zhang
Carl Yang
Jiawei Han
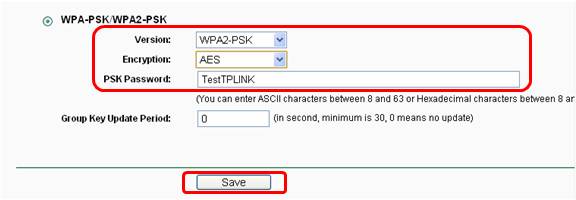
Xinwei Networks Network & Wireless Cards Driver Download For Windows Xp
arXivLabs is a framework that allows collaborators to develop and share new arXiv features directly on our website.
Both individuals and organizations that work with arXivLabs have embraced and accepted our values of openness, community, excellence, and user data privacy. arXiv is committed to these values and only works with partners that adhere to them.
Have an idea for a project that will add value for arXiv's community? Learn more about arXivLabs and how to get involved.
Abstract: Multi-view networks are broadly present in real-world applications. In themeantime, network embedding has emerged as an effective representation learningapproach for networked data. Therefore, we are motivated to study the problemof multi-view network embedding with a focus on the optimization objectivesthat are specific and important in embedding this type of network. In ourpractice of embedding real-world multi-view networks, we explicitly identifytwo such objectives, which we refer to as preservation and collaboration. Thein-depth analysis of these two objectives is discussed throughout this paper.In addition, the mvn2vec algorithms are proposed to (i) study how varied extentof preservation and collaboration can impact embedding learning and (ii)explore the feasibility of achieving better embedding quality by modeling themsimultaneously. With experiments on a series of synthetic datasets, alarge-scale internal Snapchat dataset, and two public datasets, we confirm thevalidity and importance of preservation and collaboration as two objectives formulti-view network embedding. These experiments further demonstrate that betterembedding can be obtained by simultaneously modeling the two objectives, whilenot over-complicating the model or requiring additional supervision. The codeand the processed datasets are available atthis http URL.
Submission history
From: Yu Shi [view email][v1] Fri, 19 Jan 2018 23:14:08 UTC (2,668 KB)
[v2]
Xinwei Networks Network & Wireless Cards Driver Download For Windows 7
Tue, 30 Oct 2018 20:57:12 UTC (2,668 KB)[v3]Sun, 3 Nov 2019 01:52:09 UTC (3,031 KB)
Full-text links:
Download:
Current browse context:References & Citations
DBLP - CS Bibliography
Fangqiu Han
Xinran He
Carl Yang
Jie Luo
Xinwei Networks Network & Wireless Cards Driver Download For Windows 10
Xinwei Networks Network & Wireless Cards Driver Download For Windows 8
arXivLabs is a framework that allows collaborators to develop and share new arXiv features directly on our website.
Both individuals and organizations that work with arXivLabs have embraced and accepted our values of openness, community, excellence, and user data privacy. arXiv is committed to these values and only works with partners that adhere to them.
Have an idea for a project that will add value for arXiv's community? Learn more about arXivLabs and how to get involved.
